Abstract : While working on projects that involve inference with embedding models, inference error rates were a major headache for us. We tested a few inference engines, like HuggingFace’s Text Embeddings Inference (TEI), which had a 94% error rate and Infinity Inference Engine, with a 37% error rate, both on higher context lengths (8000 tokens). Moreover, it is observed that TEI crashes when the input context length is 16000 tokens. With numbers like these, these tools weren’t ready for production deployment. So, we decided to build a new inference engine specifically to solve this problem. We called it Bud Latent, and it works. We reduced the error rate to under 1%.
Bud Latent is designed to optimize inference for embedding models in enterprise-scale deployments. Our benchmark results reveal that Bud Latent outperforms TEI by up to 90% and the Infinity by up to 85%, while also delivering a 1.4x performance boost on CPUs. With this performance and less than 1% error rate, Bud Latent is currently the most production-ready inference engine for embedding models.
We are introducing Bud Latent, a new inference engine added to the Bud Runtime stack, designed to optimize inference for embedding models in enterprise-scale deployments. Initial benchmarks indicate that Bud Latent offers up to a 90% improvement in inference performance compared to Hugging Face’s Text Embeddings Inference (TEI) and up to 85% increase compared to Infinity inference engine (torch backend), along with a 1.4x performance boost on CPUs.
Bud Latent is a production-ready inference engine with an error rate of less than 1%, compared to 94% for TEI and 37% for Infinity on higher context lengths (8000 tokens). Moreover, it is observed that TEI crashes when the input context length is 16000 tokens. The Bud Latent runtime supports a range of hardware and device architectures, including Intel, AMD CPUs, AMD ROCM, Nvidia CUDA devices, AWS Inferentia for cloud inference, and Apple Silicon & Intel Core Ultra for client-side inference. The platform also supports horizontal scaling, routing, and OpenAI-compatible APIs.
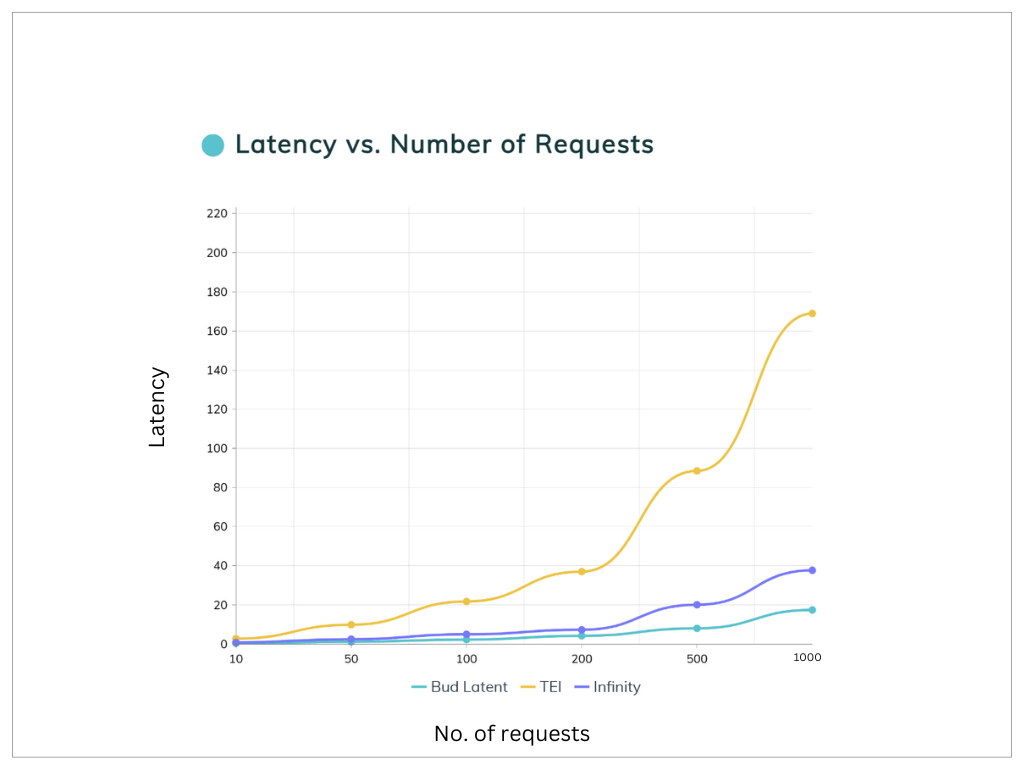
Graph 1 : Latency vs. number of requests comparison between Bud Latent, TEI and Infinity. Benchmark experiments conducted with model : gte-large-en-v1.5 on an Intel Xeon Platinum 8592V processor with 32 cores and 40GB of memory.
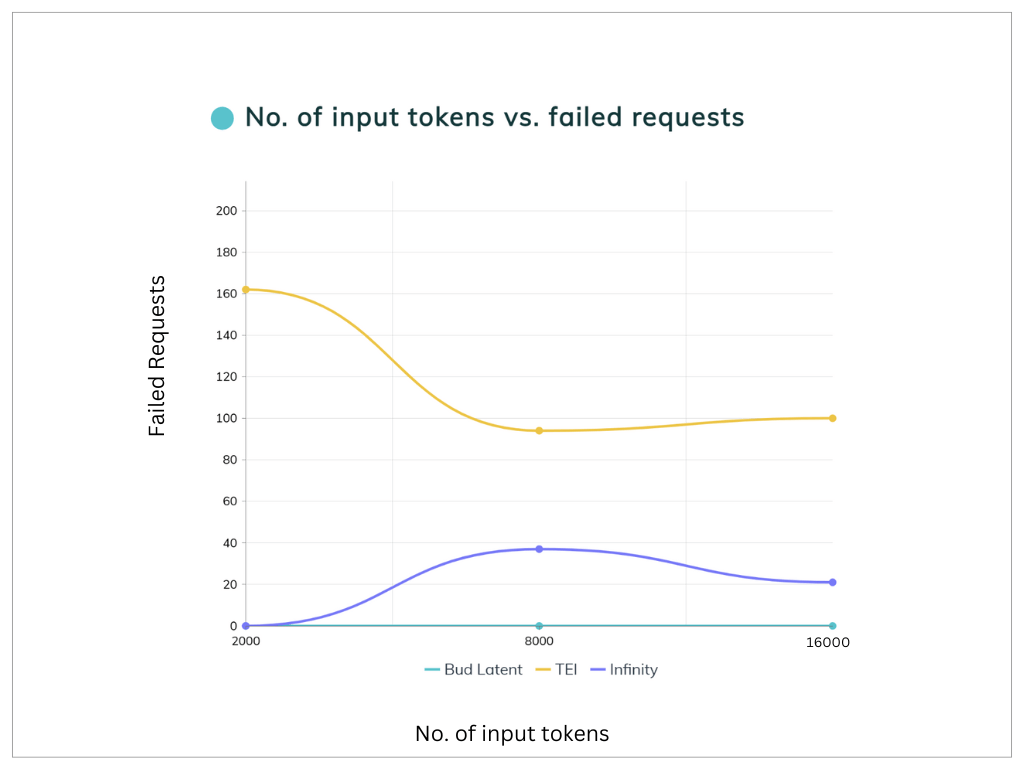
Graph 2 : Failed Requests vs. number of input tokens comparison between Bud Latent, TEI and Infinity. Benchmark experiments conducted with model : gte-large-en-v1.5 on an Intel Xeon Platinum 8592V processor with 32 cores and 40GB of memory.
The Growing Need for High-Performance Embeddings Inference
As enterprises increasingly rely on embedding models to power critical applications such as search relevance, content personalization, fraud detection, and knowledge management, the demand for robust, high-performance inference solutions has never been greater. Traditional inference engines often struggle with high error rates, high latency and cost inefficiencies, hindering real-time applications and large-scale deployments.
Bud Latent is engineered to overcome these challenges by significantly reducing error rate and latency, enhancing throughput, and minimizing infrastructure costs—all while maintaining high accuracy. The key features of the inference engine are as follows;
- State of the Art Performance: Supports Flash attention, Paged Attention, Custom optimised device specific kernels. With up to 90% performance improvement compared to TEI and 85% compared to Infinity.
- Model Compatibility: Use models straight from huggingface, modelscope or disk
- Multiplatform Support : Bud Latent offers broad compatibility across various hardware platforms, including NVIDIA CUDA, AMD ROCM, CPU, AWS INF2, and Apple MPS accelerators. This flexibility ensures that enterprises can seamlessly deploy embedding models across a range of environments, optimizing performance on each platform. It also supports heterogeneous hardware deployments, allowing you to run your embedding workload across different types of hardware.
- Production-Ready : With robust reliability—featuring an error rate of less than 1%—and scalability, the new inference engine is production-ready. It supports multi-cloud, multi-hardware horizontal & auto scaling, allowing businesses to deploy AI-powered applications at scale without compromising speed, accuracy, or cost-efficiency. Additionally, it includes support for custom performance metrics and distributed tracing with OpenTelemetry and Prometheus metrics.
- Multi-Utility: Bud Latent not only helps you create embeddings, but could also be used for reranking models, text curation models, prompt routing models, multi-modal, cross modal, text classification etc.
- Zero Configuration: Integrated with the Bud Simulator, the system automatically identifies the optimal configuration for your production deployment, ensuring it meets your Service Level Objectives (SLOs) at the lowest possible cost.
- Automated Hardware sizing & Finder: Automatically identify the right hardware across different clouds and determine the optimal hardware size to achieve the best Total Cost of Ownership while meeting your SLOs.
- Dynamic Batching and Tokenization : The engine utilizes dynamic batching and tokenization, performed by dedicated worker threads. This allows for efficient resource management and maximizes throughput by processing multiple requests simultaneously, making it ideal for high-traffic applications.
- Enhanced CPU Performance with AVX and AMX : For enhanced performance on CPU-based systems, the inference engine supports AVX (Advanced Vector Extensions) and AMX (Advanced Matrix Extensions). These optimizations combined with highly optimised custom kernels ensure that CPU resources are used to their full potential, speeding up inference processes while maintaining accuracy. Bud Latent runtime is also capable of leveraging multiple NUMA nodes available on a system for improved resource utilisation and optimal performance.
- Cloud, On-prem, BYOC and Client Deployment : The inference engine is designed to work seamlessly in both cloud and client environments, offering the flexibility to scale and deploy based on your needs. Currently, Bud Latent runtime supports 16 different cloud options and allows you to do production deployment with Kubernetes & Openshift.
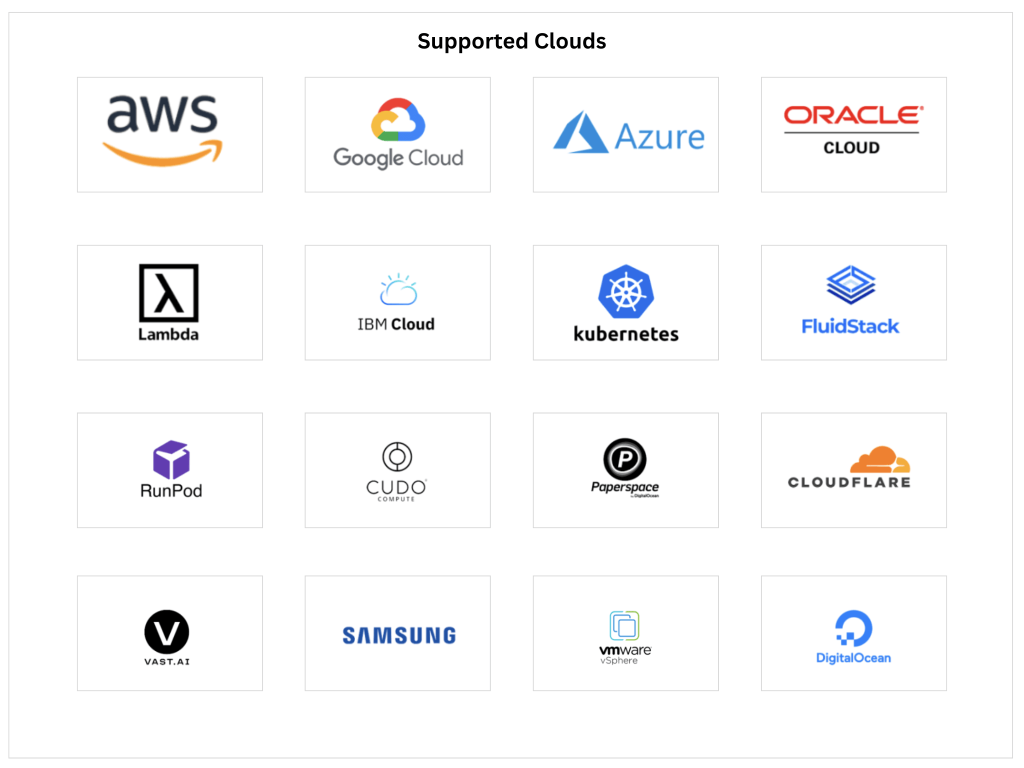
- A solution for hardware supply problem: The Bud Latent runtime enables heterogeneous cluster deployments with automated hardware finding and provisioning across 16 different cloud platforms. This ensures you can scale up or down at any time, optimizing costs while maintaining full hardware availability.
- Horizontal Scaling with Workers : Bud Runtime supports horizontal scaling via worker threads, allowing businesses to handle an increased number of requests while improving load handling. This architecture ensures that the system can scale efficiently as your application grows, maintaining optimal performance under heavy workloads.
- Int8 and FP8 Support : The inference engine supports int8 (on CPU, RCOM (AMD), Synapse (Intel Gaudis) and CUDA) and fp8 (on H100/MI300) precision for faster computations and reduced memory usage, making it possible to run large models with greater efficiency while ensuring that the results maintain the necessary precision for high-quality outputs.
- Launch Multiple Models Simultaneously : With the ability to launch and run multiple models at once, the inference engine provides flexibility in handling a variety of use cases, from search relevance and content personalization to multimodal embeddings.
- Multimodal Support : The engine supports a variety of embeddings, including text, image, and audio embeddings (Clip, Clap, Colpali), as well as reranking models. This multimodal capability enables enterprises to create more comprehensive AI applications that can process and understand a wide array of data types, unlocking new possibilities for personalized and dynamic user experiences.
The Bud Latent inference engine can be applied to a variety of use cases across multiple industries:
- AI Agents – Build high-performance AI agents with a less than 1% error rate for production-ready reliability. Create embedding-based AI agents ideal for automating workflows across various sectors such as customer service, operations management, and technical support.
- Enterprise Search & Knowledge Management – Accelerates search and retrieval of information across vast document and database repositories.
- E-commerce & Personalization – Enhances recommendation engines for dynamic, user-specific content delivery.
- Financial Services & Fraud Detection – Strengthens anomaly detection and risk analysis through real-time embedding comparisons.
- Healthcare & Life Sciences – Improves medical research and diagnostics by enabling fast similarity searches across biomedical datasets.
Bud Latent is now available for enterprises aiming to scale their embeddings-based AI applications with enhanced efficiency and accuracy. To learn more or request a demo, contact us at contact@bud.studio
Appendix : Benchmark results
Bud Latent : Up to 90% improvement in inference performance compared to TEI and up to 85% increase compared to Infinity inference engine (torch backend). Error rate of less than 1%
users | batch_size | request_rate | num_tokens | total_requests | successful_requests | failed_requests | avg_response_time | median_response_time | min_response_time | max_response_time | dtype |
10 | 1 | inf | 100 | 10 | 10 | 0 | 0.4052023692 | 0.4955194052 | 0.1907812823 | 0.500028111 | float32 |
50 | 1 | inf | 100 | 50 | 50 | 0 | 1.143477629 | 1.163029227 | 0.2575879991 | 1.600166343 | float32 |
100 | 1 | inf | 100 | 100 | 100 | 0 | 2.272522269 | 2.262270691 | 0.3166659623 | 3.241668141 | float32 |
200 | 1 | inf | 100 | 200 | 200 | 0 | 4.217472302 | 3.351719621 | 0.3354266062 | 6.649045318 | float32 |
500 | 1 | inf | 100 | 500 | 500 | 0 | 8.000135476 | 7.772920148 | 0.1859967448 | 15.03466847 | float32 |
1000 | 1 | inf | 100 | 1000 | 1000 | 0 | 17.42841425 | 17.00285405 | 0.1875152327 | 33.67127076 | float32 |
100 | 1 | inf | 500 | 100 | 100 | 0 | 8.668577559 | 9.482354475 | 0.6105506159 | 14.31473305 | float32 |
100 | 1 | inf | 2000 | 100 | 100 | 0 | 49.83315539 | 43.65044803 | 0.880711833 | 73.80447029 | float32 |
200 | 1 | inf | 2000 | 200 | 200 | 0 | 89.25694118 | 73.52233746 | 0.6558725275 | 142.813371 | float32 |
100 | 1 | inf | 8000 | 100 | 100 | 0 | 270.9934692 | 380.4362849 | 24.45675381 | 408.0344722 | float32 |
100 | 1 | inf | 16000 | 100 | 100 | 0 | 265.2299411 | 264.0894367 | 134.3735515 | 397.1633052 | float32 |
10 | 1 | inf | 100 | 10 | 10 | 0 | 0.1803239632 | 0.213504605 | 0.09858523495 | 0.217314668 | bfloat16 |
50 | 1 | inf | 100 | 50 | 50 | 0 | 0.4386955613 | 0.4266068572 | 0.2049441207 | 0.6459736768 | bfloat16 |
100 | 1 | inf | 100 | 100 | 100 | 0 | 0.8199990342 | 0.661839799 | 0.6452382393 | 1.216599256 | bfloat16 |
200 | 1 | inf | 100 | 200 | 200 | 0 | 1.448243593 | 1.385621122 | 0.3847504165 | 2.411869619 | bfloat16 |
500 | 1 | inf | 100 | 500 | 500 | 0 | 3.387109188 | 3.521169587 | 0.1180846877 | 6.130071493 | bfloat16 |
1000 | 1 | inf | 100 | 1000 | 1000 | 0 | 6.401807476 | 6.295999131 | 0.1999545451 | 12.51010011 | bfloat16 |
100 | 1 | inf | 500 | 100 | 100 | 0 | 2.812243529 | 2.443220575 | 0.360729998 | 4.807883019 | bfloat16 |
100 | 1 | inf | 2000 | 100 | 100 | 0 | 19.08222142 | 26.19665648 | 1.030380877 | 27.09224063 | bfloat16 |
200 | 1 | inf | 2000 | 200 | 200 | 0 | 28.08099131 | 25.4279731 | 0.4083833154 | 49.77493088 | bfloat16 |
100 | 1 | inf | 8000 | 100 | 100 | 0 | 149.277586 | 170.5842005 | 1.764253156 | 174.0163538 | bfloat16 |
100 | 1 | inf | 16000 | 100 | 100 | 0 | 119.0317349 | 100.3649746 | 8.976574231 | 174.0420267 | bfloat16 |
Table 1: Benchmark results. Experiments conducted with model : gte-large-en-v1.5 on an INTEL® XEON® PLATINUM 8592V processor, with 40GB of memory and 32 cores. The experiments used a data type of 32-bit floating point (float32), with an inf request rate and a batch size of 1.
Infinity : Error rate can reach up to 37%.
users | batch_size | request_rate | num_tokens | total_requests | successful_requests | failed_requests | avg_response_time | median_response_time | min_response_time | max_response_time | dtype |
10 | 1 | inf | 100 | 10 | 10 | 0 | 0.8080683077 | 0.8082979098 | 0.8058504481 | 0.809895765 | float32 |
50 | 1 | inf | 100 | 50 | 50 | 0 | 2.487429961 | 2.518104792 | 0.7012535557 | 3.380646594 | float32 |
100 | 1 | inf | 100 | 100 | 100 | 0 | 4.972873705 | 6.474263798 | 0.4868423305 | 6.986398246 | float32 |
200 | 1 | inf | 100 | 200 | 200 | 0 | 7.288230219 | 6.566767693 | 2.79964105 | 13.27286144 | float32 |
500 | 1 | inf | 100 | 500 | 500 | 0 | 20.08286735 | 18.92353356 | 0.5804256294 | 37.41144929 | float32 |
1000 | 1 | inf | 100 | 1000 | 1000 | 0 | 37.6805948 | 36.48344388 | 0.6685071178 | 72.30005198 | float32 |
100 | 1 | inf | 500 | 100 | 100 | 0 | 18.78074493 | 15.38263782 | 2.700841447 | 28.76471366 | float32 |
100 | 1 | inf | 2000 | 100 | 100 | 0 | 89.98908401 | 85.98557319 | 1.259994561 | 141.3938221 | float32 |
200 | 1 | inf | 2000 | 200 | 200 | 0 | 170.5375092 | 147.135284 | 1.66456474 | 281.7094974 | float32 |
100 | 1 | inf | 8000 | 100 | 100 | 37 | 373.602216 | 428.4562441 | 18.72330613 | 428.4682249 | float32 |
100 | 1 | inf | 16000 | 100 | 100 | 21 | 407.0072823 | 536.6985094 | 19.24124624 | 536.7168855 | float32 |
10 | 1 | inf | 100 | 10 | 10 | 0 | 0.7706522834 | 0.9086181708 | 0.4456509054 | 0.9102523811 | bfloat16 |
50 | 1 | inf | 100 | 50 | 50 | 0 | 2.242069024 | 2.942038647 | 0.6606787723 | 3.504472384 | bfloat16 |
100 | 1 | inf | 100 | 100 | 100 | 0 | 2.714340597 | 2.112246864 | 0.7078306898 | 4.373712376 | bfloat16 |
200 | 1 | inf | 100 | 200 | 200 | 0 | 5.280264955 | 5.290602886 | 1.071872769 | 9.401459731 | bfloat16 |
500 | 1 | inf | 100 | 500 | 500 | 0 | 12.40294331 | 12.52492908 | 0.5171276089 | 23.49155435 | bfloat16 |
1000 | 1 | inf | 100 | 1000 | 1000 | 0 | 24.32148973 | 24.19110726 | 0.5186605677 | 47.98807011 | bfloat16 |
100 | 1 | inf | 500 | 100 | 100 | 0 | 12.71362284 | 14.86846113 | 0.6553703807 | 16.63025535 | bfloat16 |
100 | 1 | inf | 2000 | 100 | 100 | 0 | 62.20905734 | 80.3743374 | 0.9942744263 | 80.38623325 | bfloat16 |
200 | 1 | inf | 2000 | 200 | 200 | 0 | 89.99039782 | 81.15589893 | 0.9599503074 | 157.4812552 | bfloat16 |
100 | 1 | inf | 8000 | 100 | 100 | 0 | 238.2009776 | 327.6528775 | 8.387714051 | 337.3884446 | bfloat16 |
100 | 1 | inf | 16000 | 100 | 100 | 0 | 246.364753 | 200.9632803 | 59.96540344 | 374.2751351 | bfloat16 |
Table 2: Benchmark results. Experiments conducted with model : gte-large-en-v1.5 on an INTEL® XEON® PLATINUM 8592V processor, with 40GB of memory and 32 cores. The experiments used a data type of 32-bit floating point (float32), with an inf request rate and a batch size of 1.
TEI : Error rate can reach up to 94%
users | batch_size | request_rate | num_tokens | total_requests | successful_requests | failed_requests | avg_response_time | median_response_time | min_response_time | max_response_time | dtype |
10 | 1 | inf | 100 | 10 | 10 | 0 | 2.757103388 | 2.178155268 | 0.4257001467 | 4.423851764 | float32 |
50 | 1 | inf | 100 | 50 | 50 | 0 | 9.863978257 | 10.63153264 | 0.3161862381 | 17.74905183 | float32 |
100 | 1 | inf | 100 | 100 | 100 | 0 | 21.74912045 | 21.93435366 | 0.4171652589 | 40.68083454 | float32 |
200 | 1 | inf | 100 | 200 | 200 | 0 | 37.0029104 | 34.88931386 | 0.3744490817 | 75.03766196 | float32 |
500 | 1 | inf | 100 | 500 | 500 | 0 | 88.49137289 | 89.25706704 | 0.4369468018 | 170.181461 | float32 |
1000 | 1 | inf | 100 | 1000 | 1000 | 0 | 168.9215812 | 168.7399757 | 0.4053446911 | 338.8540228 | float32 |
100 | 1 | inf | 500 | 100 | 100 | 0 | 149.4461583 | 148.2498126 | 1.432162989 | 287.6974653 | float32 |
100 | 1 | inf | 2000 | 100 | 38 | 62 | 315.9089398 | 334.5163867 | 11.35889501 | 574.5568133 | float32 |
200 | 1 | inf | 2000 | 200 | 38 | 162 | 301.1382666 | 311.5782777 | 10.11749762 | 561.5724616 | float32 |
100 | 1 | inf | 8000 | 100 | 6 | 94 | 440.917633 | 593.9873211 | 91.4583224 | 593.9925882 | float32 |
100 | 1 | inf | 16000 | 100 | 0 | 100 | 0 | 0 | 0 | 0 | float32 |
Table 3: Benchmark results. Experiments conducted with model : gte-large-en-v1.5 on an INTEL® XEON® PLATINUM 8592V processor, with 40GB of memory and 32 cores. The experiments used a data type of 32-bit floating point (float32), with an inf request rate and a batch size of 1.