NOTE: This is an ongoing research and we invite fellow researchers to collaborate on this project. If you are currently working on a related topic or have a general interest in this field and would like to collaborate on this research, please get in touch with us via this form: Research Collaboration
AI innovations have the potential to bring transformative benefits to humanity, but they also come with considerable risks. As AI technologies evolve at an unprecedented pace, several pressing questions arise: How will AI continue to shape our future? And, perhaps more critically, how should we regulate its development and deployment?
We are already witnessing a profound transformation driven by AI, but it is essential to establish governance structures to ensure that these innovations are carefully scrutinized for their potential societal impact. The challenge is not only in harnessing AI’s power but also in managing its consequences responsibly.
At present, regulatory efforts such as the European Union’s AI Act and the White House’s AI guidelines are taking initial steps toward establishing oversight. However, these frameworks remain in their infancy and are often fragmented. They are not yet comprehensive enough to tackle the full spectrum of challenges AI presents, both globally and locally.
One of the central issues is that AI, like any powerful tool, can be used for both beneficial and harmful purposes. The same algorithms that power self-driving cars, for example, can also be weaponized for cyberattacks or used to spread disinformation. To prevent AI from being a source of harm, effective regulation is essential. But how should AI be regulated, and who determines which innovations should be encouraged and which should be restricted?
In this article, we propose an Equitable Governance Framework for Balancing AI Innovation and Ethical Regulation. This framework seeks to ensure that AI’s potential is maximized for societal good while minimizing its risks.
How Should AI Be Regulated?
A fundamental rule of thumb is that if a technology causes harm, it should be regulated. However, the story is not always so simple. The impact of an innovation is rarely entirely negative or positive in the short term. In fact, history shows that technological advancements often have unintended consequences, both good and bad. Take the example of nuclear technology: the development of atomic bombs caused immense destruction during World War II. However, the same technology has since been harnessed for peaceful purposes, including energy production. If the atomic bomb had led to an outright ban of nuclear technology, we would have been denied access to the considerable benefits of nuclear power.
In a similar way, AI innovations must be treated with caution, but without stifling their potential for positive change. Like nuclear energy, AI may present risks, but its long-term benefits may outweigh the harms if appropriately regulated. Governments should adopt a measured approach—introducing regulation that minimizes the negative impacts of AI while allowing the most beneficial applications to thrive. This approach requires constant monitoring and reassessment, ensuring that AI’s potential to improve lives is maximized while mitigating its risks.
The Impact of AI on Society
The influence of AI is vast and multifaceted. Its impact can be broadly categorized into economic, social, and environmental spheres. While AI can lead to significant advances, it also presents challenges in each of these areas.
Economic Impact
AI has the potential to revolutionize the global economy, but it also raises serious concerns about job displacement and market fairness. As automation continues to advance, many industries are poised to benefit from more efficient and cost-effective operations. However, these advances also bring with them the threat of significant job losses. In particular, tasks that were once performed by humans in fields like manufacturing, customer service, and even healthcare are increasingly being taken over by AI systems. This shift has the potential to create massive unemployment unless steps are taken to retrain workers and help them transition to new roles in the AI-driven economy.
Another economic challenge is market polarization. AI-powered systems, like algorithms used by large corporations, can exacerbate inequalities by entrenching the market power of a few dominant players. These algorithms often benefit the biggest firms with the most data, creating a competitive imbalance. Furthermore, AI systems can sometimes perpetuate biases or fail to account for fair competition, potentially undermining trust in digital marketplaces and financial systems. Ensuring equitable access to AI technologies will be crucial in preventing market monopolies and ensuring fair competition.
Social Impact
On the social front, AI poses several risks that could undermine social cohesion and individual well-being. Misinformation, for example, is a growing concern. With AI-generated content becoming more sophisticated, distinguishing truth from falsehood in digital spaces is becoming increasingly difficult. AI-driven “deepfakes” and automated bots can spread harmful propaganda, sowing discord among communities and even influencing democratic processes.
Moreover, AI’s potential for hallucination—producing false or misleading outputs—is another source of anxiety. AI systems, such as generative models, have demonstrated an ability to fabricate content that appears real, but may be entirely fictional. The proliferation of such tools could contribute to the erosion of trust in information sources.
Social structures are also at risk. As AI becomes more ingrained in our lives, it can disrupt traditional social norms and relationships. People may become more isolated as they interact more with machines than with other humans. Additionally, AI could contribute to a loss of critical thinking skills and creativity. If individuals rely too heavily on AI for decision-making, there is a risk of down-skilling, where human creativity and problem-solving abilities are diminished.
AI could also lead to an increased dependency on technology, with individuals and society as a whole becoming more reliant on systems that they don’t fully understand. This dependency could make individuals vulnerable to cyber-attacks or system failures. Furthermore, as AI systems become more advanced, they may take over tasks traditionally carried out by human minds, thereby eroding our capacity to think independently.
Environmental Impact
AI’s environmental impact is another area that requires attention. While AI technologies can be leveraged to address some of the world’s most pressing environmental challenges, such as climate change and resource management, they also come with a cost. The energy consumption associated with training AI models is significant. The environmental footprint of running these technologies at scale could negate some of the benefits they provide. Responsible regulation of AI should include considerations of its ecological impact to ensure sustainable innovation.
An approach for AI regulations
Given the complexities of AI’s dual impacts, a regulatory framework should seek an equilibrium where the long-term benefits outweigh the potential harms. The framework should incorporate various factors such as the innovation’s target market, user demographics, potential adoption rates, and the environmental, social, and economic consequences. The key parameters to be considered for framing an effective AI regulation framework includes;
Market Potential and Target Demographics
Regulations should consider the specific markets in which the AI innovation will operate and the demographics it targets. Different regions, industries, and consumer groups may experience the effects of AI differently. For example, an AI tool designed for healthcare may have significant positive effects in developed countries with strong healthcare infrastructure, while the same tool could exacerbate inequalities in developing regions where access to technology and healthcare is limited.
Adoption Rates
The potential for widespread adoption of the AI innovation should be evaluated. A technology that is rapidly adopted could have both a swift positive impact, as well as a rapid escalation of negative consequences if left unchecked. Understanding the adoption trajectory helps regulators predict and mitigate adverse effects before they become widespread.
Projected Impact Evaluation
The evaluation of both positive and negative impacts must go beyond theoretical models and incorporate real-world data, when possible. This should include considerations of economic, social, and environmental dimensions. AI innovations that provide substantial economic benefits, such as creating new industries or improving existing sectors, should be balanced against the social and environmental costs, such as workforce displacement or environmental degradation.
Evaluating Release Readiness Based on Impact Scores
The regulation of AI innovations can be further refined using a formulaic approach that assesses their readiness for release based on the projected impact balance. The Impact Score (IS), a central part of this framework, is calculated as:
IS = IP − ( C * IN)
Where:
IP = The projected positive impacts of the AI innovation, such as economic growth, social benefits, or environmental improvements.
IN = The projected negative impacts, such as job loss, ethical concerns, or environmental harm.
C = A constant that adjusts for the governance framework and the severity of potential negative impacts. The value of C is qualitative, determined by the level of oversight and regulation appropriate for each specific AI innovation. It dictates how many folds of positive impact are required over the negative impacts to attain an acceptable equilibrium state for the governing body.
Based on the Impact Score, AI innovations can be categorized into three distinct pathways for release:
IS > 0: If the impact score is positive, it means that the projected benefits of the AI innovation outweigh the potential negative impacts. Such innovations can be released to the market, subject to standard regulations that ensure their responsible deployment.
IS = 0: When the impact score is neutral, it suggests that the positive and negative impacts are balanced. In such cases, a staged release approach is advisable. This involves careful monitoring and incremental deployment, ensuring that any issues related to the negative impacts are resolved before the innovation is fully integrated into society.
IS < 0: If the impact score is negative, it indicates that the AI innovation could cause more harm than good. In such cases, the innovation should not be released to the open market. Before any release, the innovation would need to be substantially reworked or abandoned if it cannot be made beneficial.
Measuring and quantifying the impact of AI innovations
We propose a novel approach that combines established methodologies to assess the penetration and impact of AI innovations in the market. Specifically, our approach integrates elements from the Diffusion of Innovation Framework, Network Models of Epidemics, and the AI Hallucination Evaluation Framework. These models provide complementary insights that, when combined, offer a comprehensive tool for forecasting both the positive and negative impacts of AI technologies across diverse demographics and geographies.
Diffusion of AI innovation
A critical aspect of any AI innovation is understanding how quickly and deeply it will penetrate the target market. AI innovations, particularly in sectors like healthcare, finance, or education, may spread rapidly, but their adoption rates can vary depending on several factors, such as technological readiness, consumer trust, and market conditions.
The Diffusion of Innovation Framework, developed by Everett Rogers, helps in assessing how new technologies spread through populations. It identifies different adopter categories—innovators, early adopters, early majority, late majority, and laggards—that define the adoption curve of a new technology. By applying this framework, we can predict the likely rate and extent of AI innovation adoption in a given market. This helps regulators estimate when and how the technology will reach critical mass and potentially begin to have widespread effects, both positive and negative.
One of the unique challenges associated with AI innovations—especially generative models like large language models—is the potential for “hallucination” or the creation of false or misleading information. This demerit of LLMs can be a major driver of negative social impacts. As these AI systems can sometimes generate content that is factually incorrect, biased, or misleading, which can have significant negative consequences when such information spreads uncontrollably.
To forecast this, we integrate the AI Hallucination Evaluation Framework, which assesses the likelihood and severity of misinformation generated by AI systems. Coupled with Network Models of Epidemics, this framework enables us to model how quickly AI-generated misinformation can spread across different demographic segments within the target market. By treating misinformation diffusion similarly to the spread of contagious diseases (as epidemics spread through populations), we can estimate how various segments of the population—such as age groups, geographic locations, or socioeconomic statuses—might be exposed to and affected by false information.
Using the SEDPNR model, we can evaluate how much fake news has penetrated the population at a given time. This model treats the diffusion of AI generated misinformation similar to how an epidemic spreads. This analogy can also be extended to evaluate how new AI innovations spread in its target market.
SEDPNR (Susceptible-Exposed-Doubtful-Positively Infected-Negatively Infected-Restrained) model take into account the necessity of an “end state” for disease transmission while considering a single trending misinformation or fake news in online digital networks. The Infected node is classified as Positively Infected and Negatively Infected based on the user’s attitude (whether the false information is viewed positively or negatively). As rumors and fake news typically spread only through solid emotions, the infected node is divided according to these sentiments. The Restrained condition refers to people who have lost interest in the knowledge over time. Those who are no longer spreaders gradually fall into the Restrained state.
This network model can also be used in conjunction with the diffusion of innovation model to assess how a particular AI innovation has penetrated the target market. The differential equations in this model can help to evaluate the rate of spread, rate of transition from different stages that includes early adopter, early majority etc.
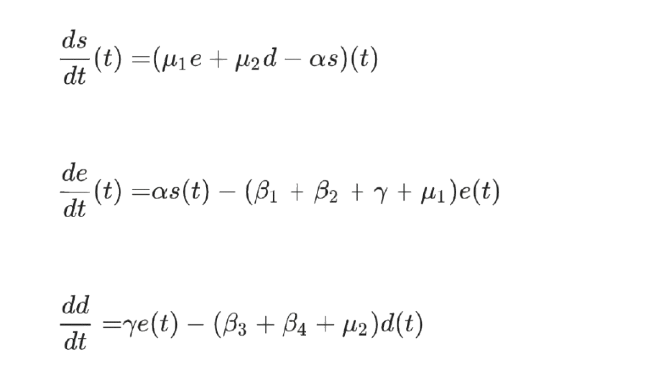
This model allows for a more precise understanding of the risk that an AI innovation poses in terms of misinformation spread, helping regulators anticipate and mitigate negative effects before they escalate.
Forecasting Positive and Negative Impacts on Affected Demographics
AI innovations can have profound effects on specific demographics, particularly vulnerable populations. For instance, job automation might disproportionately affect low-skilled workers, while algorithmic biases may worsen existing inequalities in healthcare, criminal justice, or hiring practices.
By combining the above frameworks with demographic data, we can forecast the potential negative impacts on specific groups. For example, in regions with high levels of unemployment, automation technologies may cause significant displacement, or in societies with underrepresented groups, AI systems that are not designed inclusively may perpetuate discrimination.
To quantify these impacts, we have to use a predictive model that accounts for geographic, socioeconomic, and cultural factors, enabling us to understand how deeply an innovation will affect specific segments of the population. The model also helps determine the time frame within which these negative impacts will likely unfold, guiding regulators in making timely decisions on the release of AI technologies.
While it is crucial to understand the potential risks AI innovations pose, equally important is forecasting their overall positive impact. This encompasses improvements in economic growth, social well-being, environmental sustainability, and technological progress.
To quantify these positive impacts, one has to rely on quantitative market research that takes into account projected benefits, such as job creation in emerging sectors, improvements in healthcare outcomes, or reductions in carbon emissions due to AI-powered solutions. These positive impacts must be measured in a way that accounts for both direct and indirect effects, ensuring a holistic view of how the innovation will contribute to societal goals.
By combining data on market potential, adoption rates, and demographic characteristics, we can forecast the overall positive impact AI innovations will have on the target market. This allows policymakers to gauge whether the benefits will outweigh the risks, facilitating the decision on whether an innovation is ready for release or if it needs further adjustments.
Bottom Line,
A framework to evaluate the release readiness of AI innovations using Impact Scores provides a structured and transparent method for assessing the potential benefits and risks associated with new technologies. By calculating the Impact Score, this framework offers a clear way to gauge whether the positive impacts outweigh the negative ones, guiding decision-making in the regulation and deployment of AI systems. Innovations with a positive IS can proceed with standard regulatory oversight, while those with a neutral or negative IS should undergo more rigorous scrutiny, staged releases, or even reconsideration. Ultimately, this approach fosters responsible AI deployment, ensuring that innovations benefit society while mitigating potential harms.